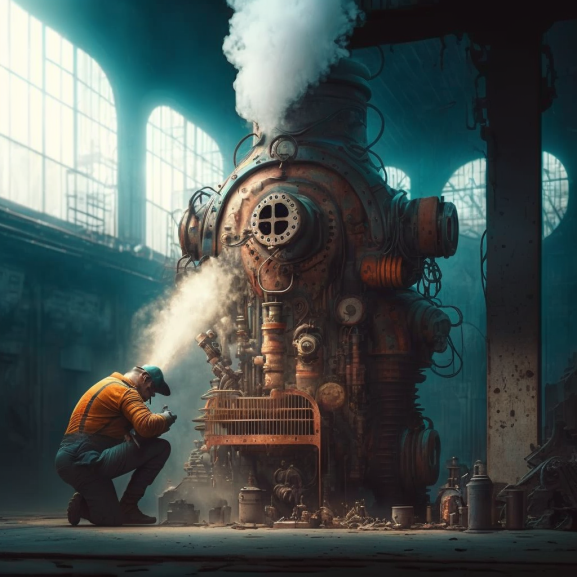
Predictive Maintenance in Logistics: Leveraging AI to Avoid Equipment Failures
Predictive maintenance is a proactive approach to maintaining equipment, in which maintenance is performed based on data-driven predictions of when equipment is likely to fail. In the field of logistics, where equipment downtime can be costly and disruptive, implementing a predictive maintenance program can help to improve efficiency and reduce the risk of unexpected failures.
To better understand the differences, here is a comparison of traditional maintenance and predictive maintenance utilizing artificial intelligence (AI).
Traditional Maintenance:
- Scheduled based on fixed intervals (e.g. every 6 months) or on the number of hours that the equipment has been in use.
- Reactive approach, performed after an issue has been identified or as a preventative measure.
- Can result in unnecessary maintenance being performed and potential overlooked failures that can lead to costly downtime.
Predictive Maintenance Utilizing AI:
- Scheduled based on data-driven predictions of when equipment is likely to fail.
- Proactive approach, performed before an issue occurs to prevent downtime.
- Utilizes sensors and machine learning algorithms to continuously monitor equipment data and predict when maintenance is needed.
- Can improve maintenance efficiency, reduce costs, and reduce the risk of unexpected equipment failures.
Here are some key points to consider when implementing a predictive maintenance program for logistics equipment:
- Predictive maintenance utilizes artificial intelligence (AI) and machine learning to analyze data from sensors on the equipment to predict when a failure is likely to occur. This allows maintenance to be scheduled at the optimal time, reducing the risk of unexpected failures and the need for reactive maintenance.
- AI can be used to analyze vibration data from sensors on the equipment to detect unusual patterns that may indicate an impending failure. One way that AI can be used for predictive maintenance in logistics equipment is through the analysis of vibration data. Sensors on the equipment can measure the vibration levels and send this data to a central system, where an AI algorithm can analyze the data to detect any unusual patterns that may indicate an impending failure. By monitoring this data continuously, the AI system can alert maintenance staff to potential issues before they become critical, allowing for timely repairs to be made.
- AI can also be used to analyze oil samples to detect contaminants or other indicators of equipment wear, allowing for timely repairs to be made before a failure occurs. Another application of AI in predictive maintenance is the analysis of oil samples to detect the presence of contaminants or other indicators of equipment wear. By regularly analyzing oil samples, it is possible to identify potential issues with the equipment before they result in a failure.
In addition to improving maintenance efficiency and reducing the risk of unexpected equipment failures, implementing a predictive maintenance program using AI can also help to reduce maintenance costs. By scheduling maintenance at the optimal time, rather than on a fixed schedule, it is possible to reduce the overall number of maintenance events and the associated labor costs.

More Practical?
Lets make follow an example based on a common warehouse conveyor system:
- Sensors are installed on the conveyor system to monitor various parameters such as vibration, temperature, and belt wear.
- The sensor data is collected and sent to a central system, where it is analyzed by an AI algorithm.
- The AI algorithm is trained to recognize patterns in the sensor data that may indicate an impending failure, such as an increase in vibration levels or an unusual temperature rise.
- If the AI algorithm detects a potential issue, it sends an alert to maintenance staff to schedule a maintenance event at the optimal time, before the issue becomes critical.
- The maintenance team performs the necessary repairs or replacements, based on the recommendations of the AI system.
- The AI system continues to monitor the sensor data and provide predictive maintenance recommendations as needed to prevent future equipment failures.
Overall, the use of AI for predictive maintenance in logistics equipment can help to improve efficiency, reduce costs, and reduce the risk of equipment failures.
By continuously monitoring equipment data and utilizing machine learning algorithms to detect potential issues, it is possible to schedule maintenance at the optimal time and keep equipment running smoothly.
#lastmile #lastmiledelivery #onlinegrocery #delivery #supplychain #grocerydelivery #ecommerce #customerexperience #sustainability #technology #darkstores #localretailers #predictiveanalytics